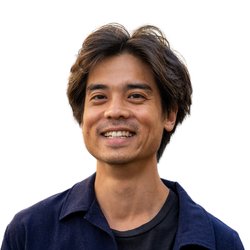
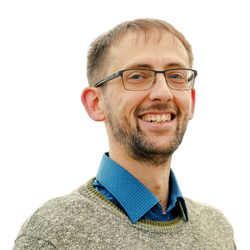
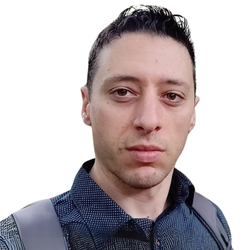
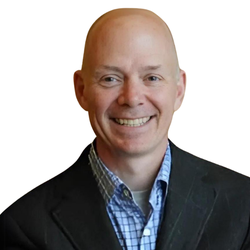
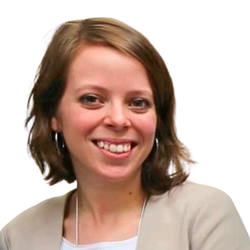
Advancements and Challenges in Causal Inference and Personalized Treatment
A Talk by Thomas Debray PhD , Dr. Janie Coulombe , Dr. Orestis Efthimiou , Prof. Dr. Robert Platt and Dr. Long Nguyen
Register To Watch This Content
This Talk has ended, but you can still watch the replay! Register now to get access.
About this Talk
Join Thomas Debray, Prof. R.W. Platt, and Dr. L. Nguyen for an engaging panel discussion featuring guest speakers Dr. Janie Coulombe and Dr. Orestis Efthimiou. This session will delve into innovative statistical methodologies for personalized treatment and causal inference, providing a preview of topics from the panelists’ forthcoming book.
Dr. Coulombe will address the complexities of analyzing longitudinal data with irregular, covariate-dependent visit times, highlighting methods to improve causal estimates from observational data. Dr. Efthimiou will discuss evidence-synthesis approaches for developing and validating models that predict treatment effects at the patient level by combining data from multiple clinical trials.
In this interactive session, the panelists will explore both theoretical advancements and practical challenges, setting the stage for a deeper dive into their upcoming book on personalized healthcare analytics.
Agenda:
Intro & Key Principles of Causal Inference in RWE Studies
- Robert Platt, and Long Nguyen
Addressing irregular and covariate-dependent visit times in longitudinal studies using observational data for causal inference
Longitudinal observational data are increasingly used to draw causal inferences, and a substantial body of literature now exists on causal estimators for longitudinal data. However, a challenge that is often overlooked in medical and epidemiological literature is the irregular timing of patient observations. Often, the timing of patient visits (or measurement) varies based on individual patient characteristics—a phenomenon we refer to as covariate-dependent visit times. This irregularity can introduce bias into standard causal estimators, making it an important issue that requires careful consideration.
In this talk, we focus on the average treatment effect as a target parameter of interest. We will review the statistical methods proposed to address covariate-dependent visit times, including imputation techniques and inverse weighting strategies, highlighting their respective advantages and limitations. If time allows, we will discuss R software packages available for implementing these approaches.
Evidence synthesis methods for developing and validating models for personalized treatment
Medical interventions often have varied effects on different patients, yet most randomized clinical trials (RCTs) are designed to detect average, rather than individualized, treatment benefits. As a result, estimating patient-level treatment effects using data from a single RCT is challenging, given that RCTs are not typically powered to uncover personalized responses. Combining data from multiple RCTs provides a powerful approach to explore heterogeneity in treatment effects and increases statistical power. In this talk, we will discuss some recent advancements in methods for predicting patient-level treatment effects between two or more interventions using evidence synthesis. By aggregating data from multiple trials, we can more effectively identify patient subgroups that may respond differently to treatments and better understand the variability in outcomes. This approach offers opportunities for more personalized healthcare strategies. Additionally, we will examine how real-world data may potentially complement analyses of RCT data. Finally, we will outline various methods for validating models that predict treatment effects at the individual patient level. Model validation is essential for ensuring the reliability of these predictions in clinical practice. Methods described in this presentation will be illustrated with real clinical examples.