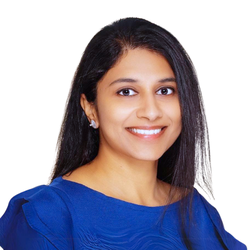
Statistical Innovation in the Cambrian Age of Biotech
A Talk by Manjari Narayan PhD (Fellow, Speculative Technologies)
About this Talk
In the past decade, statistics and causal machine learning have made tremendous theoretical and methodological advances in tackling problems of validity that arise in health and medicine. In parallel, we are living in a new Cambrian age of biotechnology —- incredible new high throughput screening technologies that can test millions of biological perturbations, novel model systems to emulate human biology, dizzying measurement technologies at many resolutions to create next-generation biomarkers and frontier AI models such as alpha fold have made formidable advances in predicting protein structure and function. There is great enthusiasm and optimism that all these advances will make it easier, cheaper, and faster to predict and optimize when next-generation therapies are likely to work in humans and to tailor therapeutic interventions to individuals.
However, these emerging technologies have generated new kinds of challenges for statistical and causal inference. Scientific collaborators often associate statistical thinking with classical experimental design, power analysis, or improving the reliability of measurements or regression modeling. Identifying novel problems of validity, or better assessment of generalizability by asking different quantitative questions and re-designing studies to answer them can be challenging to pursue. I will share what I have learned from other disciplines about why use-inspired statistical innovation is often a public good that needs alternative institutions beyond academia and industry. Statistical scientists can join the growing movement to create new R&D institutions to create tools and capabilities that might not otherwise exist. I will conclude with lessons I’ve learned since joining this movement.