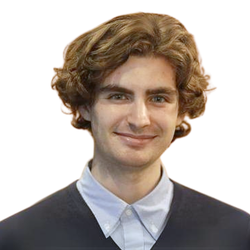
Considering health equity in medical prognosis: What makes a model fair?
A Talk by Jose Benitez-Aurioles (Health Data PhD Student, University of Manchester)
About this Talk
There are increasing concerns about the fairness of clinical prediction models, particularly those leveraging machine learning, across sensitive attributes, such as ethnicity or gender. However, it is unclear how statisticians should consider equity when designing, training, and validating models. While a sizeable body of work has developed around algorithmic fairness in the artificial intelligence field, most guidance either focuses on data collection and model deployment or is not apt for tackling healthcare-specific challenges.
We propose assessing the fairness of predictions through a model-agnostic approach that leverages principles of health equity, decision curve analysis, and net benefit (NB) to quantify and compare the clinical impact of models in each subgroup. We extend the formula for the NB to allow for subgroup comparisons, through an additional inequality term, and account for the value of well-calibrated models through a weighted area under a rescaled decision curve. By measuring the NB across subgroups, developers can better understand how the introduction of their model benefits the most underserved populations, as well as check whether their model narrows or widens health inequalities. In addition, we show how the concept of distributive justice emerges in situations of limited resources.
This work improves our understanding of how predictive modelling is placed within a wider health equity framework.