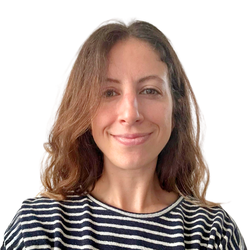
Leveraging Survival Analysis and Machine Learning for Repeated Events in Cancer Surgery: A User-Friendly R Toolkit
A Talk by Juliette Murris (Biostatistician, Pierre Fabre)
About this Talk
Random survival forests are very useful in the prediction of the time to the first hospital readmission and in modeling the effects of predictors on survival at assumptions. Their use consequently constantly increasing in medical research. However, the clinical interest may rely on the multiple readmissions patients undergo. As a result, we have adapted these forests to survival data with multiple event occurrences, referred to as recurrent events, in the presence or absence of a fatal event. This work aims to introduce the use of RecForest to help understand the burden of disease after cancer surgery. Our approach is in five steps, to i) discern the relevance of multiple and fatal events, ii) develop trees and build the forest, iii) thoroughly evaluate performance, iv) provide the importance of variables, and v) enable predictions on new data. Data from hospital readmission in metastatic colorectal cancer patients were used to illustrate our purpose.